N. (Nima) Monshizadeh Naini, Prof
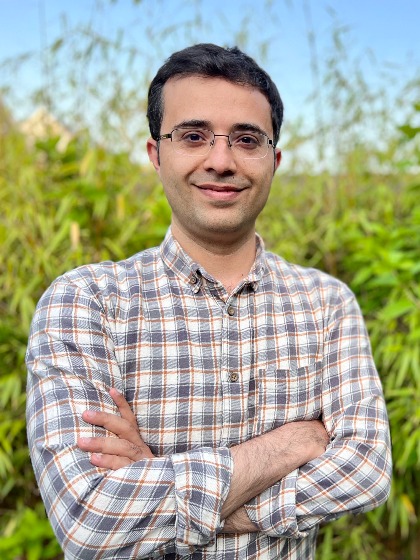
Research interests
Nima Monshizadeh's core research area concerns Optimization and Control of Cyber-Physical Human Systems (CPHS). Conventionally, humans are viewed as independent entities that consume, use, or operate cyber-physical systems. However, this conventional view is changing, and humans are expected to play a much more active role in those systems, resulting in fully intertwined complex cyber-physical human systems (CPHS). Currently, within this theme, two research lines pursued in Dr. Monshizadeh research group :
I. Coordination of self-interested users
Individuals, firms, and parties make decisions that not only affect their own well-being but also affect the entire society as a whole. As these decisions are primarily based on individual benefits, their aggregate effect can be in sharp contrast with the interest or safety of the overall society, and may even lead to catastrophic events like power blackouts and market crashes. The resulting devastating societal effects underscore the necessity of proper coordination among self-interested agents. The aim of the research group along this line is to reduce the gap between self-interested decisions and those aligned with the welfare of society. Dynamic information design , dynamic pricing, and game-theoretic market mechanisms are used as the main tools to achive this goal. Main applications of interest are power systems and traffic networks.
II. Privacy-aware control and optimization.
Modern cyber-physical human systems include several components interacting with each other to achieve a certain goal. The extensive data collection and information exchange raise urgent privacy issues that need to be carefully addressed. Motivated by the rising privacy concerns in the society, the research group of Dr. Monshizadeh develops privacy-preserving optimization and control algorithms in cyber-physical human systems. Two general approaches within this research line are: (i) Tailoring and adapting the existing powerful privacy-preserving tools (e.g. differential privacy and encryption) to the needs and setups of CP(H)S. The main feature/twist here is the fact data is not static and is generated by a dynamical system modelled by differential/difference equations. (ii) The second approach is to develop new privacy-preserving methods that are tailored for a given control or optimization problem. The main advantage would be to keep the system performance level (unlike noise addition schemes) and to provide light computations (unlike encryption schemes).