Synthetic data speed up yeast research
The growth and division of budding yeast are often studied using time-lapse microscopy. Artificial intelligence systems are extremely useful in this area, as they can automatically detect and follow changes in individual cells via time-lapse microscopy video monitoring. However, training an AI system requires lots of manually annotated data, which can take many months to generate. Scientists at the University of Groningen have shown that synthetic data can be used to train a convolutional neural network in a matter of days, rather than months. The new system performs as well as the best available neural networks for the detection of yeast cells.
Budding yeast is one of the best studied organisms in the world. Yeast cells serve as a model for more complex cells (such as human cells) in the study of fundamental processes. However, there are still a lot of things we do not know about them, explains assistant professor in computational biology Andreas Milias-Argeitis: ’An important unanswered question is how yeast cells control their growth during their cell cycle. In other words, how is biomass accumulation coordinated with the replication of DNA, the synthesis of essential building blocks, and the division process?’
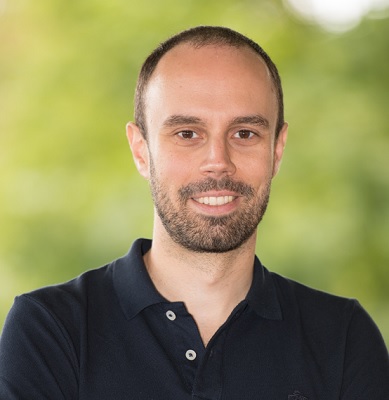
Training
Milias-Argeitis, whose research interests lie at the interface of biology and computation, studies these questions using a large volume of time-lapse microscopy data. ’We observe how hundreds of individual cells grow and divide over many generations while monitoring key readouts via microscopy. We are also able to perturb the cells, for example by switching certain genetic pathways off using light, a technique that is called optogenetics.’ By following single cells through the series of time-lapse images, he then observes how perturbations affect the phenotype of the cell. However, these experiments produce huge amounts of data and, therefore, some kind of automated image analysis is needed. Convolutional Neural Networks (CNNs) are well suited to this task but these powerful systems need training to enable them to recognize the cells.
‘This is achieved by providing the CNN with many annotated microscopy images in which someone has marked the outlines of thousands of cells,’ explains Milias-Argeitis. Annotation has to be perfect and, therefore, it is a very time-consuming task. ‘A student might take months to provide a good, annotated set of training data.’ And if the research question changes, the training programme has to start again. A faster way to train the CNN would really speed up research.
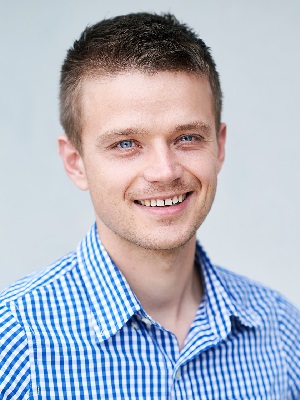
Synthetic data
So, when the University of Groningen Center for Information Technology (CIT) put out a call for proposals on data science, Milias-Argeitis pitched the question of whether a more efficient training system could be devised. His proposal was accepted by the CIT and he was teamed up with data scientist Herbert Kruitbosch. They started working on an idea of Kruitbosch: a training method that would use synthetic data, rather than annotated real data. Milias-Argeitis: ‘The use of synthetic data for AI training purposes has, so far, been very limited in biology. However, Herbert is extremely experienced in image processing and has a keen eye for picking out essential cell features, even though – or perhaps because – he is not a yeast specialist.’
Kruitbosch generated a dataset with yeast-like shapes and tweaked settings for e.g. cell deformation, size, and noise. The synthetic dataset was then used to train a convolutional neural network called Mask R-CNN for image processing. The trained CNN was subsequently presented with its first real yeast sequences. ‘And it worked surprisingly well. In fact, I couldn’t believe it was true when he showed me the first results.’ The new system was eventually tested against a state-of-the-art convolutional neural network trained with annotated real data and it turned out that it worked just as well. The major advantage of the synthetic data is that a training set can be generated in a day. Furthermore, redesigning the system for a different use takes just a few days. And it is very user-friendly; it takes a couple of hours to learn how to work with it.
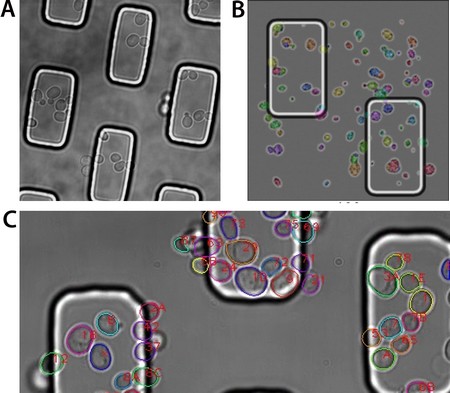
Specific events
‘All of this means that our experiments can be designed and performed a lot faster,’ says Milias-Argeitis. ‘Since the trained network works without any user input, it can even be used for real-time data analysis linked to changes in cell behaviour during an experiment. For example, we can now perform a microscopy experiment in which we determine the locations and identities of individual cells, measure their responses to an optogenetic perturbation, and adapt the optogenetic input specifically for each cell.’
The project with the CIT has now been completed, the final results were published in the journal Bioinformatics on 10 December 2021, and all software and algorithms are available in a public code repository for anyone to use. ‘We are now working on further developments of the AI system, for example teaching it how to detect specific events during cell division, or mutant cells with different morphologies. We hope that we can do most of it ourselves, but we are happy to have Herbert as an advisor.’
Reference: Herbert Kruitbosch, Yasmin Mzayek, Sara Omlor, Paolo Guerra and Andreas Milias-Argeitis: A convolutional neural network for segmentation of yeast cells without manual training annotations. Bioinformatics, 10 December 2021
Software and algortims are available in this public repository
Last modified: | 27 June 2024 3.51 p.m. |
More news
-
17 July 2024
Veni-grants for ten researchers in Groningen
The Dutch Research Council (NWO) has awarded a Veni grant of up to €320,000 each to ten researchers of the University of Groningen and the UMCG. The Veni grants are designed for outstanding researchers who have recently gained a PhD.
-
15 July 2024
Funding for RUG researchers from National Growth Fund programme Circular Plastics NL
For research on making plastics circular, Professors Patrizio Raffa and Katja Loos together receive about 1.2 million euros from the National Growth Fund programme Circular Plastics NL.
-
09 July 2024
NWO Open Technology funding for improving quality 3D printing technology
Dr. Liangliang Cheng receives a NWO OPT grant of EUR 950,000 for research on safer application of metals in 3D printing technology.