Research Digest: Advancing Inclusive Text-to-Speech for Low-Resource Languages
Date: | 10 April 2025 |
Author: | Phat Do |
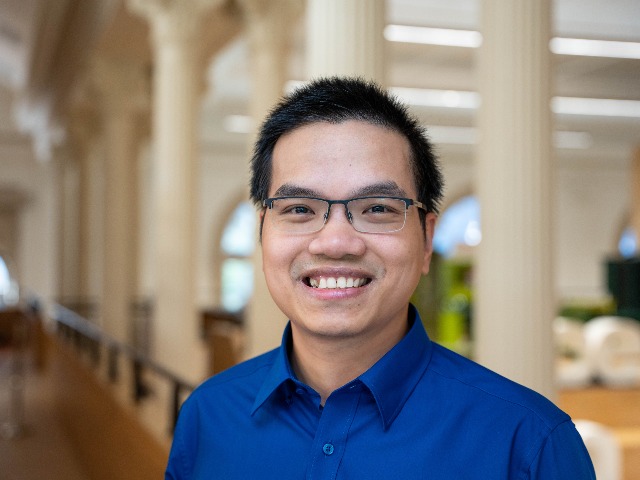
How can we make high-quality speech synthesis accessible to all the world’s languages, not just a privileged few? This blog piece examines the progress and limitations of current Text-to-Speech (TTS) technology, especially for low-resource languages (LRLs). It presents cross-lingual transfer learning as a promising solution, illustrated through a case study on Frisian, and offers practical strategies for building inclusive TTS systems that don’t compromise on quality. Read on to dive into the topic!
The Rise of TTS and Its Limitations
Speech synthesis is the generation of artificial speech, also usually called Text-to-Speech synthesis (TTS) since the input is text in most cases. Applications of TTS include, but are not limited to, the speech responses in human-machine interaction (e.g., that with virtual assistants such as Apple Siri, Google Assistant, and ChatGPT), accessibility tools (e.g., web and screen readers), and language learning aid (e.g., pronunciation samples on Duolingo). As such applications are increasingly popular, TTS is playing an increasingly important role.
Thanks to rapid advancements in deep learning and associated hardware in recent years, state-of-the-art (SOTA) TTS systems can be said to have approached, if not reached, the human-level in terms of speech quality. Though this is only under certain evaluation conditions (e.g., TTS is prone to unnatural speech prosody for long utterances) and proper TTS evaluation is a whole research sub-field, this is to say that TTS research has largely reached a satisfactory level in terms of synthetic speech quality. At least, that is the case for the most common settings in TTS research: testing with (American) English or Mandarin Chinese, using hundreds or thousands of hours of training data.
Tackling Low-Resource Language Challenges
Data is mentioned here to highlight a discrepancy between two aspects of TTS research: quality and inclusivity. Like other fields using deep learning-based approaches, SOTA TTS systems rely on large amounts of high-quality training data to deliver their impressively human-like speech quality. For TTS, the standard training data is recordings by professional speakers, done with good quality recording equipment, free from background noise, and annotated accurately. Commercial TTS systems may use hundreds or even thousands of hours of training data, while academic research, though less demanding, still usually requires at least dozens of hours. Such requirements mean it can be prohibitively expensive to obtain sufficient training data for TTS in a new language. Consequently, the progress from SOTA TTS advancements has benefited primarily only a small number of the world’s languages, leaving the remainder, i.e., the majority, relying on older TTS technology with lower quality, or even having no access to TTS at all. In other words, while TTS research is doing well in terms of quality, it still has a lot to do regarding inclusivity. Languages without sufficient available training data for TTS, or language technology in general, can be referred to as low-resource languages (LRLs).
Though not simple to reach an exact number, roughly 98.5% of the approximately 7,000 languages in the world can be considered LRLs. It should be noted that resource availability has less to do with a language’s number of speakers, and more to do with its commercial potential, given the dominance of tech corporations in the field. In this day and age, LRLs face severe challenges in terms of language policies aiming at promoting minority languages and encouraging linguistic diversity, as well as preserving and revitalizing endangered languages. This calls for an innovative approach to TTS for LRLs, one that eases up the data requirements while retaining the speech quality. One such approach is cross-lingual transfer learning: pre-training the TTS model on a “source language” (a high-resource language) before fine-tuning it on the “target language” (the LRL). The model can then make use of what it has learned from the source language’s ample data to “transfer” to the target language, thereby requiring less LRL data to learn from. Though cross-lingual transfer learning has been explored before, questions about its “best practices” remain. For example, for a given target language, what is the most suitable (i.e., giving the best speech quality) source language to transfer from? Intuitively, it may be one from the same language family, but is this a good general criterion? Or is there one that is more straightforward yet more effective? Another question is, since the source and target languages mostly likely have different TTS inputs (e.g., different alphabets or phones—the smallest unit of speech sounds), how to deal with this mismatch problem while maintaining efficiency?
Case Study and Future Directions
In my PhD project, I empirically investigated the questions above, together with a few more research questions that revolve around the optimal implementation of TTS for LRLs. With the internal support from Campus Fryslân and the tremendous help from the Frisian Academy, I worked on Frisian as the main case study in the experiments. For selecting the source language, I compared several criteria based on often-used language family classifications with a novel measure that quantifies the similarity between any two given languages’ phone systems, and found that the latter outperformed the former in almost all test scenarios. This means anyone looking at building TTS for a certain LRL can use this measure as a reference to effectively decide on the source language, potentially saving resources. For the input mismatch problem, I addressed it in two ways. First, I proposed a novel “phone mapping” method to automatically map all phones in the target language to their closest counterparts in the source language, thereby increasing the transfer learning efficiency. Second, I validated the use of universal phonological features (descriptions of how a phone is articulated) instead of characters or phone labels as input to TTS. Experiment results showed that both approaches improved the synthesized speech quality. Beyond Frisian, I also extended the experiments to a wide and diverse range of LRLs (Bulgarian, Georgian, Kazakh, Swahili, Urdu, and Uzbek) and found that the findings still held for most test scenarios. Some test utterances from these experiments can be found at phat-do.github.io/transfer-SSW23. To demonstrate the above research findings more straightforwardly and accessibly, I trained and deployed two open-source Frisian TTS models publicly at huggingface.co/spaces/phatdo/Frysk-TTS. One is trained on roughly 32 hours of multi-speaker public data from the Frisian subset of Mozilla Common Voice, while the other is trained only on 20 minutes of Frisian data (after pre-training on 14 hours of public Dutch data). For transcribing the input text into phones, the models use the G2P Frysk model and script kindly provided by the Frisian Academy (accessible at fa.knaw.nl/fa-apps/graph2phon/). While there is no official evaluation, the fine-tuned model does quite well considering its limited Frisian training data. I invite everyone to give the models a try! They are not supposed to be complete TTS products, so you will likely notice some issues, but I look forward to your feedback so I can keep improving them. If this text interests you, please feel free to reach out anytime if you want to know more about my research goal of making high-quality TTS accessible to all varieties of languages (so, not just low-resource languages, but also language varieties, dialects, etc.). Inclusive speech technology is also a major theme in the MSc. Speech Technology programme at Campus Fryslân.
About the author
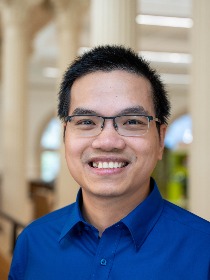
Phat Do is finalizing his PhD research in Text-to-Speech (TTS) synthesis for low-resource languages (LRLs) at the Speech Tech Lab (Center for Innovation, Technology and Ethics) at Campus Fryslân, University of Groningen. He is supervised by Dr. Matt Coler (Speech Tech Lab), Dr. Jelske Dijkstra (Frisian Academy), and Dr. Esther Klabbers (phAIstos Speech & Language Technology Services). His research interests also include speech recognition for LRLs, expressive and controllable TTS, code-switching in TTS, and lightweight on-device TTS.