Open Source Science for Human-Machine Interaction: Helping machines understand and produce non-literal speech in multilingual contexts.
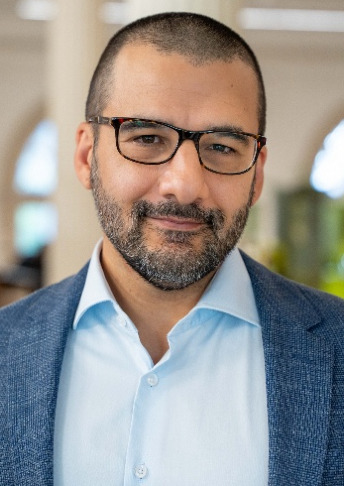
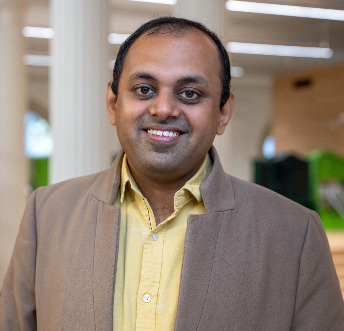
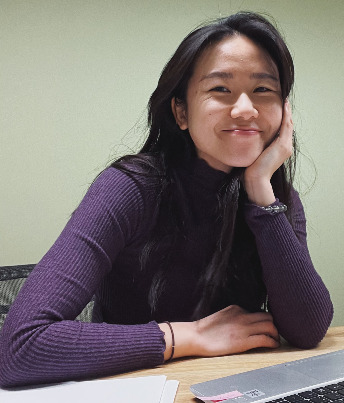
Open Research objectives/practices
-
Making research outputs freely accessible
-
Using online tools to increase transparency of research processes
-
Using open collaborative methods to increase efficiency and widen participation
-
Developing open educational materials
-
Promoting and facilitating Open Research practices
Introduction
Our project develops SarcEmotiq, an open-source, multilingual tool for detecting sarcasm in speech across cultural and linguistic contexts. As human-machine interaction (HMI) becomes prevalent, understanding nuanced communication, like sarcasm, is critical for natural interfaces. AI assistants like Siri or Alexa struggle with non-literal language, creating a significant gap between human-human and human-machine communication. SarcEmotiq addresses this by focusing on the complexities of sarcasm detection, paving the way for context-aware AI systems that respond better to subtle human cues.
SarcEmotiq also has applications in assistive technologies, aiding individuals with neurodegenerative conditions or those on the autism spectrum who may struggle with interpreting sarcasm.
By making SarcEmotiq open-source and multilingual, we foster international collaboration to advance HMI. This project contributes to more natural human-centered AI interactions and opens up new possibilities for cross-cultural communication studies and the development of inclusive technologies.
Motivation
-
Strategic OA publishing: We've embraced open-access publishing to maximize tour research's impact. Our recent papers are published OA [1,2], but some are not [3], as we strategically opted not to pay high processing fees, reserving the OA budget for others not covered by the Taverne agreement.
-
OS software: SarcEmotiq, our primary deliverable, is open-source and available on GitHub (https://github.com/x-y-g/SarcEmotiq), promoting transparency and collaborative improvement.
-
Open data: We've made our datasets (https://osf.io/buvq5/) public, allowing others to build on our work.
-
Preregistration: In the next phase, we will pre-register our study designs on OSF.io, allowing us to distinguish between confirmatory and exploratory analyses, increasing reproducibility.
-
Open collaboration: Our project has fostered international collaboration,such as with Dr. Nagendra Kumar's lab at IIT Indore, resulting in our joint work [4].
-
Open education plans: We are developing Jupyter notebook tutorials on sarcasm detection, freely available on GitHub, supporting wide access and modification by educators and researchers, including the MSc Voice Technology program at the University of Groningen. Our goal is to create hands-on learning tools for students and professionals in speech analysis and NLP.
Lessons learned
-
Need for speech-driven sarcasm detection: Despite advancements, services like Siri and Alexa remain limited. Our research highlights the gap in detecting non-literal expressions like sarcasm. By sharing our work, we aim to improve HMI systems.
-
Importance of open-source research: More open-source research is crucial in advancing speech-driven sarcasm detection. While text-based sarcasm detection is well-studied, speech-based detection has received less attention. Our open approach addresses this gap and encourages collaboration.
-
Impact on pathology training: Through open dissemination of our findings, we've realized the potential of our tools in pathology training, particularly for improving social integration of individuals with neurodegenerative conditions. This unexpected application demonstrates the value of open science in fostering interdisciplinary connections.
-
Challenges of explainable AI: While deep learning has enabled powerful models, many remain opaque. In developing SarcEmotiq, we've prioritized explainable AI. By sharing our methodologies grounded in linguistic theory, we contribute to trustworthy AI systems.
-
Benefits of open collaboration: Our open approach has facilitated collaborations with linguists and cognitive scientists, enhancing the quality and applicability of our research.
-
Balancing openness and ethics: We've learned to navigate the challenges of sharing speech data while respecting privacy concerns, leading to best practices for anonymizing speech data in open-source projects.
-
Community engagement: By sharing our progress and challenges, we've fostered a community of researchers and practitioners interested in speech-based sarcasm detection. Their feedback has led to new applications and continuous improvements to SarcEmotiq.
URLs, references and further information
[1]. Gao, X., Nayak, S., Coler, M. (2022) Deep CNN-based Inductive Transfer Learning for Sarcasm Detection in Speech. Proc. Interspeech 2022, 2323-2327, doi: 10.21437/Interspeech.2022-11323.
[2]. Li, Z., Gao, X., Zhang, Y., Nayak, S., & Coler, M. (2024). A Functional Trade-off between Prosodic and Semantic Cues in Conveying Sarcasm. In Proceedings of Interspeech 2024 (pp. 1070-1074). ISCA. https://doi.org/10.21437/interspeech. 2024-1962
[3]. Gao, X., Nayak, S. and Coler, M., 2024, May. Improving sarcasm detection from speech and text through attention-based fusion exploiting the interplay of emotions and sentiments. In Proceedings of Meetings on Acoustics (Vol. 54, No. 1, p. 060002). Acoustical Society of America. https://doi.org/10.1121/2.0001918.
[4]. Gao, X., Shubhi, S., Gowda, K., Li, Z., Nayak, S., Kumar, N., Coler, M. (Manuscript submitted for publication) AMuSeD: An Attentive Deep Neural Network for Multimodal Sarcasm Detection Incorporating Bi-modal Data Augmentation.
OS Github: https://github.com/x-y-g/SarcEmotiq
OS dataset: https://osf.io/buvq5/
Last modified: | 06 January 2025 12.14 p.m. |