AI Governance: The Why and How
Datum: | 03 mei 2024 |
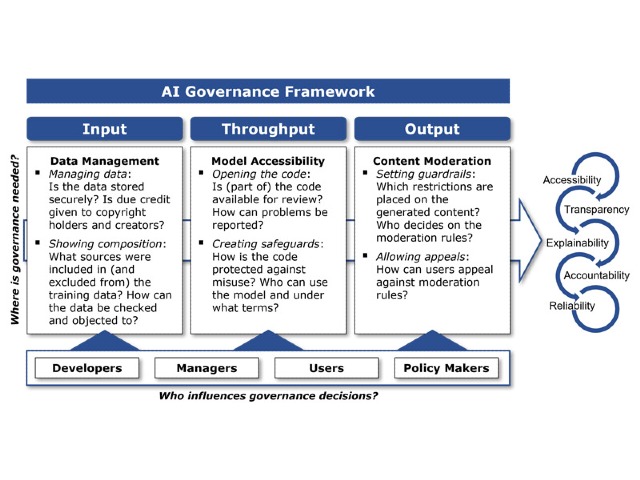
Ensuring safe and transparent artificial intelligence (AI) through an appropriate governance framework is a critical concern in both the development and deployment of this technology. Designing and using AI without such governance is like building a high-performance car without a steering wheel. In this blog post, I argue that AI governance not only makes AI better, but can also help organizations gain and maintain a competitive advantage.
AI governance involves managing data, developing models and monitoring deployment. These activities are critical to ensuring quality, safety, and compliance in AI applications. As users rely on AI for a growing range of activities, from speeding up routine tasks to enhancing creative processes, robust governance frameworks are indispensable in mitigating risks such as biased outcomes, the proliferation of misinformation, and other detrimental consequences. Specifically, AI governance requires the consideration of three critical phases:
1.Input Governance: This phase focuses on acquiring and managing data ethically and securely. Robust input governance ensures data quality, safe storage, and compliance with regulations, essential for maintaining trust and credibility. For example, healthcare providers utilizing AI must adhere to privacy regulations such as GDPA and HIPAA to safeguard patient data. Additionally, individuals and organizations should have the option to remove their data from the models, even if it is publicly available. While companies are sometimes reluctant to fully disclose their input data used for training to avoid imitation and legal liabilities, a lack of transparency in the methods of data collection, processing, storing, and sharing can cast doubt on the ethical justifiability of the entire model. For example, The New York Times filed a lawsuit against OpenAI and Microsoft, alleging copyright infringement of its training data used for ChatGPT.
2.Throughput Governance: Here, the emphasis lies on the development and maintenance of AI models. Effective throughput governance involves processes for model development, validation, and bias mitigation. For instance, financial institutions using AI for credit scoring must ensure fairness and transparency in their models to maintain trust and avoid discriminatory practices. A straightforward way to improve transparency in throughput is by releasing the model as open-source code for anyone to review, similar to what Meta did with the publication of its large language model LLaMA 2 through the open-source platform Hugging Face. While this openness may heighten the risk of imitation, it also nurtures the growth of a broad ecosystem, offering firms opportunities for complementary applications and potential revenue streams from commercial use of the code through license fees.
3.Output Governance: This phase involves monitoring model performance and integrating AI outputs into decision-making processes. Robust output governance ensures the reliability and relevance of AI-driven outputs. For example, e-commerce platforms leveraging AI for recommendations must continually assess and adjust algorithms based on user feedback to remain competitive. For generative AI models, establishing guardrails is especially crucial and challenging. While unrestricted freedom may lead to abuse, excessive regulation can stifle creativity, freedom of expression, or the adoption of beneficial uses. A case in point is Google Gemini’s AI image generator that responded to a prompt to generate images of 1943 German soldiers by outputting racially diverse Nazis, clearly overstepping the mark in its output guardrails.
Comprehensive AI governance is crucial for effectively leveraging AI and maintaining a competitive edge. By strategically managing inputs, throughputs, and outputs, developers and organizations can improve data integrity, mitigate risk, and foster trust among stakeholders while remaining competitive. A crucial trade-off centers on transparency, which can enhance the reliability and acceptance of AI applications, yet simultaneously intensify competition through easier imitation. By investing in governance frameworks and improvement processes, organizations can ensure accessibility, transparency, explainability, accountability, and reliability while minimizing the risks of biased results, hallucinations, misinformation, and legal liability. Assessing the governance practices and identifying areas for improvement will become increasingly central to firms seeking to remain competitive in the AI race.
Author: Marvin Hanisch - m.hanisch@rug.nl
Reference:
Hanisch, M., Goldsby, C.M., Fabian, N.E., & Oehmischen, J (2023). Digital Governance: A Conceptual Framework and Research Agenda. Journal of Business Research, 162, Article 113777, 1-13. https://doi.org/10.1016/j.jbusres.2023.113777