Christos Emmanouilidis | Faces of FEB
The Faculty of Economics and Business is a faculty with a great diversity of people who all have an impact, big or small, on science and society. But who are these people? Within ‘Faces of FEB’ we connect with different students, staff members and researchers of the faculty and give a little peek into their world. This month: Christos Emmanouilidis, an associate professor whose current research and teaching methods revolve around AI-based decision making.
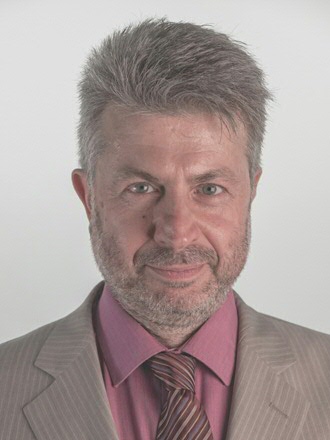
1. Can you tell us a bit about yourself?
I am Christos Emmanouilidis and I work at the intersection between Engineering, Computing and Industrial Management. I joined the Operations Department of FEB in the autumn of 2020. Carrying over 20 years of experience from Industry, Academia, Research & Innovation and Government positions, it intrigues me to see problems from the different perspectives of the knowledge and innovation triangle. Enterprises, the knowledge production sector (both Universities and Research Institutions) and Governments (and individuals working there) see different challenges and mean different things, depending on the stakeholder viewpoint you take. I have also been involved in standardization committees, for example in ISO and CEN, focusing on maintenance and asset management (that is physical engineering and not financial assets), which is of interest as standards distil the converged view of such stakeholders on specific domains.
2. Much of your current research revolves around AI-based decision-making, why did you decide to study this topic?
When I first worked with artificial neural networks, the motivation came from a problem which had to be solved: how to control a biochemical process, widely used for municipal wastewater treatment. Although such neural networks, but also current AI, are perceived as sufficiently able to act in a completely data-driven manner, the practice is somewhat different. Outcomes can be influenced by design, knowledge integration, interaction, system implementation choices and activities which are not determined at a single instance in time. These have dynamics which evolve. So I looked upon the blending of human and domain knowledge, but also the emergent properties of synergies between technical / physical systems, AI and humans. This included taking a look at genetic algorithms and evolutionary computing, control and recommender systems, but also fuzzy logic and neurofuzzy computing in the past. But whichever problem it was the target, whether it was from my main domain of interest, that of industrial maintenance and asset management, all the way to other domains which included culture and tourism, energy, or even medical applications, there was a common theme: ignore the domain knowledge and expertise at your peril.
And it’s not just in decision making. AI is about the cognitive functions that we associate with thinking and acting as humans: perception and situation awareness, recognition and categorisation, monitoring and prediction, memory and learning, interaction and communication, reasoning, and eventually problem solving and action execution. There is an interplay between such functions which is dynamic in nature. This is explored in our European Project STAR (star-ai.eu) and the Human-Centric AI approach we take there for Manufacturing Industry. In one example, the interplay between human-systems-AI is in the form of Active Learning, that enables AI, human, and eventually the system as a whole to benefit from synergies and the final outcome to be more than the sum of its parts.
3. What do you want to teach students about AI, and why?
The interest remains in problem solving. I would love to be able to think together with students and jointly show that AI outcomes, though they may appear as coming out of magic at times, they are actually influenced by choices we make. So adopting a problem-based learning approach, it is interesting to see students exploring problems, and making choices of methods, and possible solutions, becoming increasingly confident along the way that they can break into the perceived magic of AI to conceive, design, implement, and eventually manage solutions involving AI: not just talk about AI but do it.
For example, in the Data Mining and its Applications course, students embark on a journey that helps them to internalise related concepts and methods, and apply them in practice through a Data Analytics platform. The students are empowered to create their own data workflows, seeking to produce solutions to individual problems. In the Smart Industry Operations course, which we designed only two years ago, we deal with the basic building blocks of Smart Industry. We specifically delve into aspects of the interplay between humans, systems, and AI. The journey that students make goes beyond using methods for decisions but allowing students to think about further concepts which at first appear as abstract, but in the context of AI can become very practical and pragmatic, such as the concepts of bias management, ethics, and the added value of Explainable AI. How for example specific choices we make regarding data, methods, algorithms, the way we take decisions, or even more about how we interact with AI, can tilt an outcome one way or another and students work with Python programming to explore and apply in practice how such abstract concepts can tangibly be integrated into solutions.
4. In 10 years, how much do you think the world has changed due to AI-based decision-making?
AI has seen several waves of evolution, disillusionment, further breakthroughs and so on. But while in the past it was more of a niche subject, now everybody realizes that it is not just here to stay, but it already shapes the present and will shape even more the future. What was computationally prohibitive in the past, now it is not. And in the future it will be even less so. What was difficult to integrate in the past, now with connectivity being pervasive, it’s not. Irrespective of the extent to which we are aware of it or not, what we do, perceive, judge, decide or act upon is already influenced by AI somewhere in the loop. It is pointless to lament for changing or lost jobs: other technology revolutions in the past changed them too. What is different now is that the impact is beyond physical: it’s cognitive. AI can and will increasingly produce outcomes which we tend to associate with cognitive functions. A unique characteristic of AI-driven systems is that these are systems that learn over time. They learn by implicit or explicit examples. Connectivity makes the examples upon which AI can learn even more ubiquitously available.
A well-known fact in the past was that when data-driven software tools were offered to you for free, it was because the source of the data was you. Project it further and you see that the learning example for AI is also you. If we don’t like the AI outcomes, this is probably because we don’t like much of what we as humans do, when providing examples. I don’t want to trivialize moral arguments, but we need to take on board practical lessons so that we can influence AI outcomes. While arguably the last 10 years have made AI mainstream, we need to think and act upon how we steer AI towards outcomes we collectively and/or individually value as worth achieving.
Last modified: | 04 May 2023 09.37 a.m. |