Beyond Numbers: Why Data Science Needs Interdisciplinary Thinking
Date: | 27 November 2024 |
Author: | Maya Nolles |
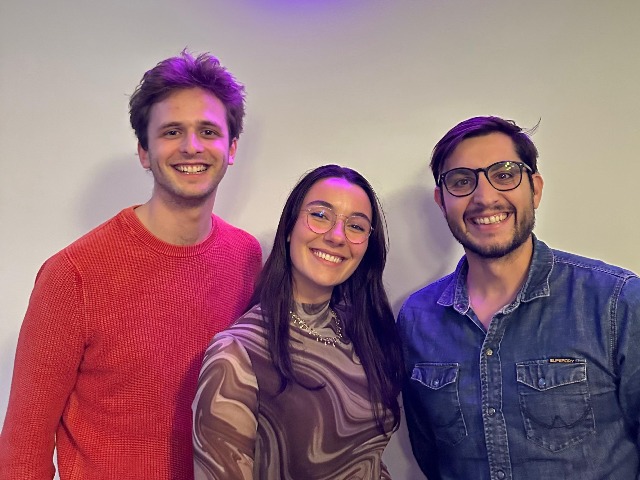
Hi there, I’m Maya! Two years ago, I graduated with a BSc. in Global Responsibility and Leadership from Campus Fryslân, and now, I'm pursuing a Master’s in Earth Sciences at Vrije Universiteit Amsterdam. During my bachelor’s, I developed a strong interest in data science and also coordinated the development of the Data Science & Society webclass. Reflecting on my experience at CF, and considering how I currently use data, I wanted to explain why I believe interdisciplinary data science degrees, like Data Science & Society, hold tremendous value compared to traditional data-focused programmes.
What Exactly is Data Science?
Let’s start with a quick overview. Data science involves extracting meaningful insights from data to answer questions, inform decisions, or drive innovation. It blends key concepts from mathematics and statistics, computer science (including artificial intelligence), and the specific domain knowledge relevant to the data being analyzed. This fusion makes data science inherently interdisciplinary. To truly understand what data means and derive valuable insights, you need more than programming and algorithms; you need a solid grasp of the context the data comes from.
The Shortcomings of Traditional Data Science Programmes
Many data science programmes focus heavily on programming, statistics, and the logical underpinnings of data analysis, while often overlooking the importance of domain expertise. However, this approach misses a crucial piece of the puzzle: the ethical, social, and practical implications of data. Without the necessary domain knowledge and broader social perspective, things can go wrong.
Take, for example, the Dutch “Toeslagenaffaire” scandal, in which an algorithm mistakenly flagged thousands of families as potential fraud cases based on flawed assumptions, resulting in severe financial and emotional distress. Another instance is showcased in the film Coded Bias, which highlights how flaws in facial recognition technology can lead to biased outcomes across various domains, from hiring practices to healthcare, and even the criminal justice system. These examples demonstrate that data science is about more than just technical proficiency. Without an understanding of the ethical, societal, and philosophical implications of our analyses, data science can inadvertently cause harm.
❝Without an understanding of the ethical, societal, and philosophical implications of our analyses, data science can inadvertently cause harm.❞
The Need for Interdisciplinary Data Science Education
This is where programmes like Data Science & Society step in. Such degrees intentionally combine data science with a wider lens that includes ethics, governance, and philosophy. By doing so, they encourage students to view data science in a broader context, one that considers not just technical requirements but also the societal impacts of data-driven decisions.
This approach is essential to prevent the kinds of biases and harms that have already occurred. When data scientists are trained to think critically about the broader effects of their work, they are better equipped to develop solutions that are both innovative and responsible.
My Data Science Journey: From Programming to Earth Sciences
Although I’m still a student, I've had the opportunity to apply data science to a number of projects, many of which go beyond the typical coding challenges. My primary interest lies in the intersection of data science and geoscience. This means I work with data related to the Earth, analyzing everything from climate patterns to resource distribution and geological phenomena.
Each project has taught me that data science in this field isn’t just about running algorithms or visualizing data; it's about understanding the implications for the environment and society. For example, when studying climate data, I don’t just want to know the numerical trends but also the socio-environmental impact of these patterns. How might they affect vulnerable populations? What kind of policies could mitigate potential negative outcomes?
Why Data Science & Society Matters for the Future
As data continues to shape industries and influence every part of our lives, the importance of responsible, context-driven data science cannot be overstated. Programmes like Data Science & Society equip students with not only the technical skills but also the critical thinking necessary to ask the right questions and consider the ethical implications of their analyses. This holistic view ensures that data science serves as a tool for positive change, rather than a source of unintended harm.
❝Programmes like Data Science & Society equip students with not only the technical skills, but also the critical thinking necessary to ask the right questions and consider the ethical implications of their analyses.❞
Choosing an interdisciplinary path in data science might seem unconventional, but it’s exactly what’s needed in today’s world. Data science isn't just about numbers or algorithms; it's about people, communities, and the way our work shapes society. And that, to me, is why it’s so valuable. So if you're considering a data science career, I urge you to think beyond traditional programmes and explore interdisciplinary options. In a field as impactful as this, understanding the broader implications is not just beneficial—it’s essential.
How do our Data Science & Society students use their interdisciplinary skills to create meaningful impact? Take a peek below!
About the author
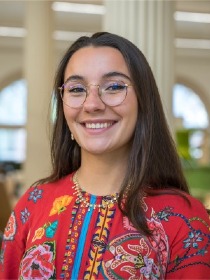
Maya Nolles is a graduate from the BSc. in Global Responsibility & Leadership who coordinated the development of the Data Science & Society web classes. Maya is currently pursuing a Master’s in Earth Sciences at the Vrije Universteit Amsterdam, where she focuses on Earth observation research, connecting science to real-world applications.